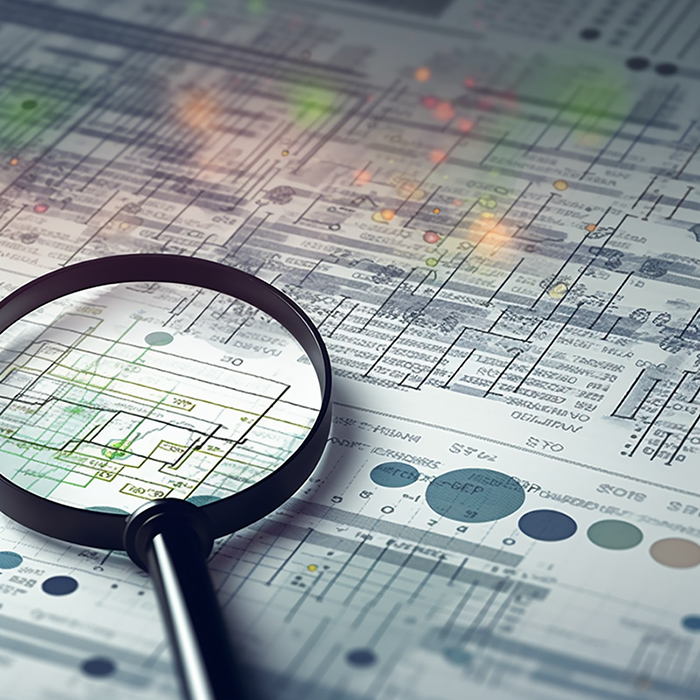
What Are The Benefits of Semantic SEO Research?
Semantic analysis is understanding the meaning and context of natural language, such as words, phrases, and sentences.
Semantic SEO and analysis help to create quality content, often by writing content semantically related to keywords grouped into topics rather than keywords.
Semantic SEO (research) is important for online marketing because it helps search engines understand your content better and match it with the user’s intent and needs. It also helps you create more engaging and informative quality content that provides value to your audience and builds trust and authority.
Some of the ways successful semantic search SEO works together with other SEO to improve search engine rankings and user experience are:
- Optimize your content for topics, not just keywords, by covering all the subtopics and related keywords and concepts that users might search for.
- Answer “People Also Ask” questions in the search results, which can increase your visibility and click-through rate.
- Use structured data and schema markup to provide rich information that enhances your snippets and makes them more attractive and clickable.
- Leverage natural language processing and machine learning to create content that matches the user’s tone, language, and preferences.
- Use entity-based optimization to link your content to other relevant entities (people, places, things) that users might be interested in.
History of Semantic Search
Semantic search (mentioned earlier) is a term that describes how search engines try to understand the meaning and context of a user’s query and provide the user’s search intent with most relevant and useful results. Semantic search has evolved as search engines (read: GOOGLE) have become more sophisticated and capable of processing natural language and complex queries. Here is a brief history of how semantic search work:
– In 2012, Google introduced the Knowledge Graph, a database of information about real-world entities and their relationships. The Knowledge Graph was Google’s first step in developing the importance of entities and context over strings of semantic keywords.
– In 2013, Google rolled out the Hummingbird update, a major overhaul of its core algorithm. Hummingbird was designed to better understand the intent and meaning behind a user’s search query, especially for conversational queries and long-tail queries.
– In 2015, Google announced RankBrain, a machine-learning system that helps Google process and then rank pages in search results. RankBrain uses artificial intelligence to learn from user behavior and feedback and to interpret queries that Google’s algorithm has never seen before.
– In 2019, Google launched BERT, a neural network-based technique for natural language processing. BERT stands for Bidirectional Encoder Representations from Transformers, and it helps Google understand the nuances and context of words in a query. BERT can also handle prepositions, which are often important for understanding the meaning of a query.
Semantic search is not only a search engine algorithm or limited to Google. Other major search engines like Bing, Yahoo, and DuckDuckGo also use semantic search techniques for better user results. Semantic search is also used in other domains, such as e-commerce, social media, and voice assistants.
Semantic search is important for SEO because it affects how users find and interact with web pages and with your content. By understanding how semantic search works and what influences it, you can optimize your content for user intent, relevance, and other high-quality content. You can also provide rich information through semantic writing that helps search engines better understand your content and its context.
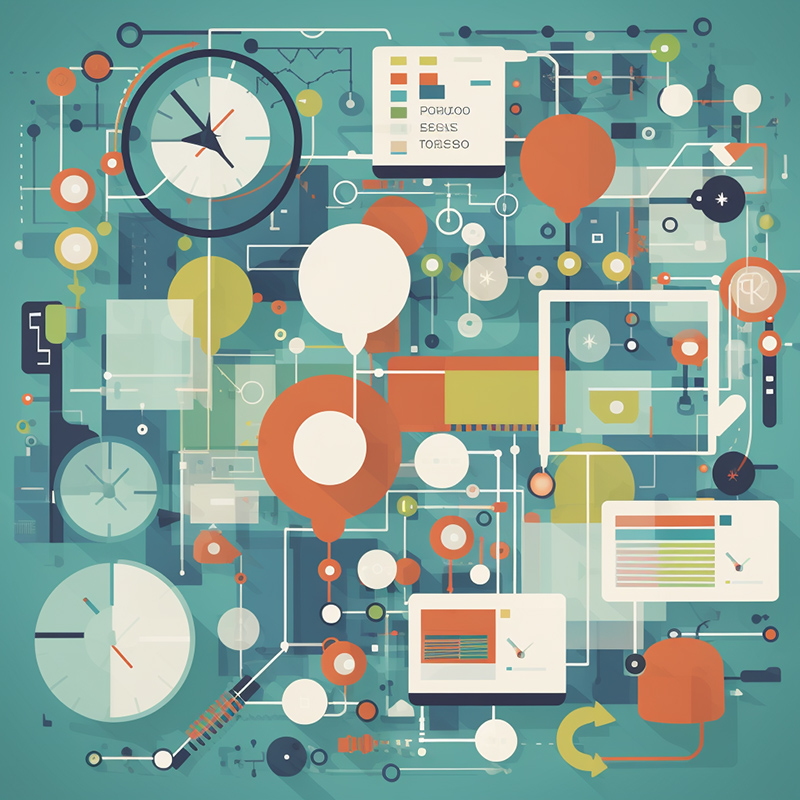
How NLP Enhances Google’s Search Result Grading and Ranking
Semantic search is a collection of features that improve the quality of search results by understanding the user’s intent and the contextual meaning of terms as they appear in the searchable data space. Semantic search uses natural language processing and machine learning techniques to analyze the query and the relevant content, and to find the most relevant and useful matches.
Semantic search can help optimize content for different queries and niches by providing more interactive and responsive search engine results pages for user searches. For example, a Google Search can:
- Provide direct answers to questions that users may ask, such as “What is the capital of France?” or “How many calories are in an apple?”
- Extract captions and highlights from documents that best summarize the content, with key passages marked for easy scanning.
- Use concept matching and synonyms to find related terms and topics that users may be interested in, such as “car” and “automobile” or “SEO” and “search engine optimization.”
- Understand the intent behind ambiguous or complex queries, such as “best pizza near me” or “How to fix a leaky faucet.”
- Rank results based on semantic relevance, not just keyword matching, by considering user location, preferences, behavior, and feedback.
Leverage semantic search for content or page optimization
- Write content in a natural and conversational tone, using clear and simple language that users can understand.
- Focus on the user’s intent and needs, not just on keywords or phrases.
- Provide accurate and up-to-date information that answers common questions or problems that users may have
- Use structured data and metadata to help search engines understand the content and its context.
- Include relevant images, videos, links, and other multimedia elements to enrich the in-depth content and provide more value to users
How Does Semantic Search Answer a Searchers Question?
Semantic search works by using natural language processing and machine learning to analyze the user’s query and the content on the web page and to find the most relevant and useful results based on the user’s own search intent, and the query context. Semantic search also considers other factors, such as the user’s location, search history, preferences, and feedback, to provide personalized and accurate results.
Semantic search aims to understand natural language the way a human would and to provide answers to questions that users may ask. Semantic search distinguishes between different entities (people, places, things) and their relationships and uses structured data and metadata to help search engines better understand the content and its context.
Some examples of how the semantic search engine works are:
– If you ask Google “What is the largest mammal?” and then follow up with “How big is it?”, Google’s search engine will understand that “it” refers to the largest mammal (a blue whale) and provide a direct answer with a featured snippet.
– If you search for “best pizza near me,” Google will use your location and other factors to provide local results with ratings, reviews, directions, and contact information.
– If you search for “car,” Google will use concept matching and synonyms to find related terms and topics that you may be interested in, such internal links to multiple keywords such as “automobile,” “vehicle,” “auto,” “car insurance,” “car rental,” etc..
– If you search for “Barack Obama,” Google will use its Knowledge Graph to provide information about the entity, such as his biography, occupation, family, achievements, etc.
Semantic search is not only limited to Google. Other search engines like Bing, Yahoo, and DuckDuckGo also use semantic search techniques to provide better user results. Semantic search is also used in other domains, such as e-commerce, social search bar, media, and voice search assistants.
Semantic analysis tools
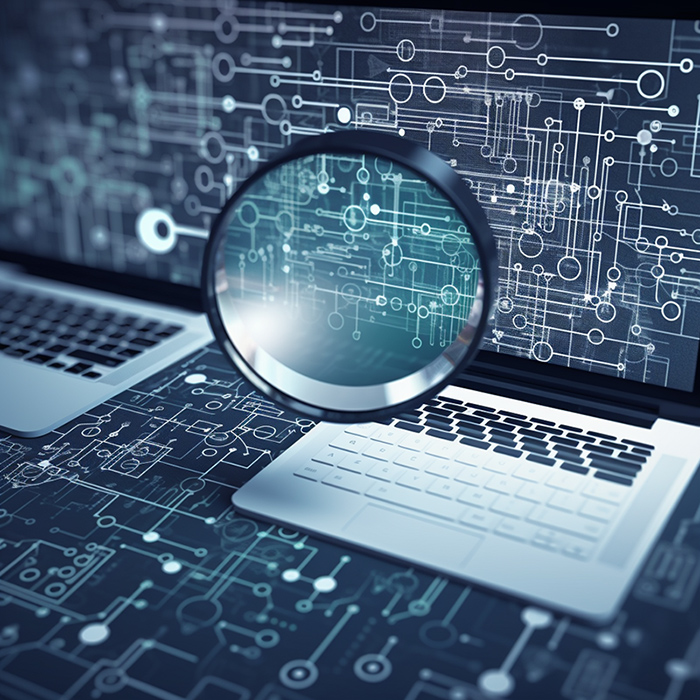
Semantic analysis draws meaning, analyzes text grammatical structure and identifies relationships between words in a particular context. It is an essential sub-task of natural language processing and the driving force behind machine learning tools like chatbots, search engines, and text analysis.
Semantic analysis tools are software applications that use artificial intelligence to automatically analyze the textual data of a website and understand what keywords, expressions, and topics are relevant for search engine users. This is in the SEO realm, but also useful for writers to cover topics comprehensively and understand search queries holistically. Some of the best tools available for semantic web analysis are:
Here is a brief overview of how you can use each tool:
- Surfer: Surfer is a cloud-based on-page optimization tool that allows you to analyze and compare your own pages against what currently ranks on the SERPs¹. You can use Surfer’s Content Editor to create or edit your content based on the guidelines provided by Surfer, such as word count, keywords usage, headings structure, media elements, etc¹. You can also use Surfer’s SERP Analyzer to get a detailed breakdown of the on-page factors that influence the ranking of each page for your target keyword.
- Ahrefs: Ahrefs is a comprehensive SEO tool that covers various aspects of SEO, such as keyword research, backlink analysis, site audit, rank tracking, etc. You can use Ahrefs’ Keyword Explorer to find relevant and low-competition keywords for your content based on semantic search intent³. You can also use Ahrefs’ Site Explorer to research your competitors’ organic traffic, backlinks, and paid ads strategies.
- Marketmuse: Marketmus is an AI-powered content marketing platform that helps you easily plan, write and optimize long-form content. You can use Marketmus’ Content Brief to generate a detailed outline for your content based on your target keyword and audience. You can also use Marketmus’ Content Editor to write or edit your content with the help of AI suggestions, such as headlines, subheadings, paragraphs, images, etc.
- SEO Quantum: This SEO solution integrates a semantic crawler, keyword strategy, and semantic analysis tool to help you optimize your website’s content. It suggests ways to improve your content’s structure, relevance, and readability.
- TextOptimizer: This tool analyzes and compares your text with top search results, providing suggestions to enrich your content with relevant terms and phrases. It helps you write better content for your target audience and rank higher on Google.
- SemRush: As a market dominator, SemRush offers a comprehensive suite of tools for keyword research, competitor analysis, content marketing, and more. It helps you get keyword rankings, discover your niche’s most popular topics and questions, analyze content performance, and optimize it for SEO and readability.
- YourText.Guru: This solution analyzes your text and gives feedback on improving its semantic coherence, lexical diversity, and readability. It provides a list of keywords and expressions to use in your content and evaluates content quality. It helps you create optimized content for SEO and user experience.
- Define your goals and target audience before creating or optimizing your content.
- Use these tools to complement your research and creativity, not as a substitute.
- Experiment with different tools and compare their results to find the best fit for your needs.
- Monitor your content performance using analytics tools such as Google Analytics or SemRush.
- Keep updating your content regularly based on user feedback and search trends.
Semantic analysis skills
Semantic analysis skills are needed to apply semantic analysis to SEO. Semantic analysis is understanding natural language (text) by extracting insightful information such as context, emotions, and sentiments from unstructured and structured data markup together. It gives computers and systems the ability to understand, interpret, and derive meanings from sentences, paragraphs, reports, or any document of a similar kind.
Key concepts involved in semantic analysis are:
Semantic mapping: A technique that visualizes the relationships between a text’s words, keywords and phrases, concepts, and topics. It helps to identify a text’s main themes, keywords, and subtopics and how they are connected².
Semantic coding: A technique that assigns codes or labels to different text segments based on their meaning and relevance. It helps to organize and categorize text data for further analysis and interpretation.
Natural language processing (NLP): A branch of artificial intelligence that deals with the interaction between computers and human language. It involves various tasks such as speech recognition, natural language understanding, natural language generation, sentiment, latent semantic analysis, and more.
Machine learning: A branch of artificial intelligence that involves creating systems that can learn from data and make predictions or decisions without being explicitly programmed. It involves various techniques such as supervised learning, unsupervised learning, reinforcement learning, deep learning, and more.
Artificial intelligence (AI): A broad term that refers to machine simulation of human intelligence processes. It involves various fields such as computer vision, natural language processing, machine learning, robotics, and more.
Skills needed to perform semantic analysis and apply SEO:
Analytical skills: The ability to collect, process, and interpret large amounts of text data using various tools and techniques. It also involves identifying patterns, trends, and insights from text data and communicating them effectively.
Linguistic skills: Understanding natural language’s structure, meaning, and usage in different contexts and domains. It also involves applying linguistic rules and principles to text data and identifying ambiguities, nuances, and subtleties in human language.
Technical skills: Using various software applications, programming languages, frameworks, and libraries that enable semantic analysis. Some common semantic analysis tools are Python, R, NLTK, spaCy, Gensim, TensorFlow, PyTorch, Scikit-learn, MonkeyLearn, etc.
SEO skills: The ability to optimize website content for search engines by using semantic analysis techniques. It involves understanding search queries, conducting keyword research, competitor seo and semantic analysis skills, and content marketing using semantic analysis tools. It also involves measuring and improving website performance using analytics tools such as Google Analytics or SemRush⁴ to create a great SEO strategy.
Some resources and courses for learning more about semantic analysis are:
- Semantic Analysis: Definition, Why Use It, And Best Tools In 2021: A blog post that explains what semantic analysis is, why it is important, and some of the best tools available for semantic analysis in 2021.
- Step By Step Guide To Mastering NLP – Semantic Analysis: A blog post that explains what semantic analysis is, how it works, what some of the techniques involved in semantic analysis are, and how to implement them using Python.
- Semantic Features Analysis Definition, Examples, Applications: A blog post that explains what semantic features analysis is, what are some of the examples of semantic features analysis, and what are some of the applications of semantic features analysis in 2022.
- Natural Language Processing Specialization: A Coursera specialization that covers various topics related to natural language processing such as sentiment analysis, text summarization, machine translation, speech recognition, etc.
- Natural Language Processing with Python: A book introducing natural language processing using Python and NLTK. It covers various topics such as tokenization, tagging, parsing, classification, extraction, etc.