In other posts I’ve described how to find levels of association from a core topic to the sub-topics you should cover to have thorough and holistic content coverage on your website. We do this because Google wants your site to “be an expert” on the niche it covers, and meeting their criteria is a crucial part of ranking high. But, we also shoot for comprehensive content because users want to find all answers related to the topic that brought them to you. This post discussing why the techniques are so accurate.
With the announcement of RankBrain‘s tremendous success, we have further evidence that Google is leveraging machine learning more heavily for ranking signals. RankBrain is their system for answering completely unique queries, and it operates under the umbrella of the Hummingbird Semantic algorithm. Move over Author Rank and other grand speculations about the most important ranking factors in years to come: Google is an AI powerhouse and they are clearly increasing the confidence they have in using this to provide better search results.
Most SEOs believe their machine learning will be super powerful in understanding the relationship between off-site links. Links are still the most important rank signal. They will remain critical “votes of confidence.” How can Google know if a link to your site is in a guest post you wrote? Probably pattern detection via machine. I digress.
In short, content gaps are found by comparing the phrases appearing in your Google Search Console (a.k.a.: Webmaster Tools) account. Are the core topics below the parent theme of your site found in words you rank top 100 on Google? The software Surfer SEO is long overdue as a reasonable priced tool that lets all SEOs quickly find topic relationships and gaps.
How Mobile Devices and Voice Recognition Forced Search Engines to Pivot
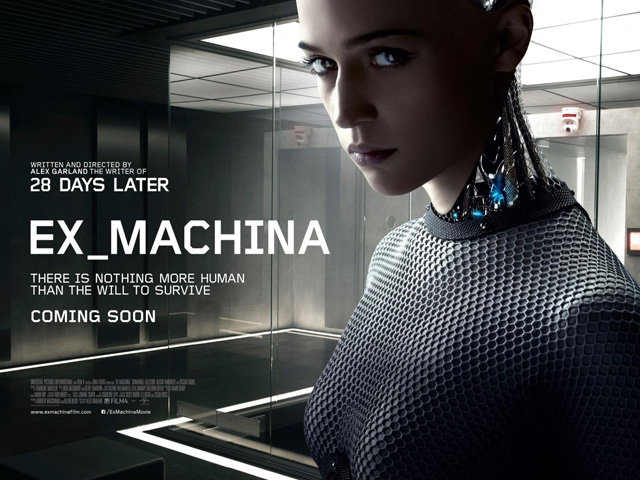
Make no mistake; Hummingbird is good for both users and webmasters. As users gravitate further to mobile devices, they rely on search engines for increasing numbers of long-tail queries. Some searches are only seen by search engines once in four months. Mobile devices are for use “on the go.” People need quick input of their search and quick results; they do not want to type eight-word queries via a tiny keypad. They turn to voice recognition to speed the process with complex queries. When we use speech recognition technology, we tend to form the search in a more conversational structure. Matching different ways of saying the same thing necessitates Google use machine learning to understand user needs. Mobile also tends to bias social networks. People are the authorities on Twitter and Facebook, so trust becomes a natural extension of the natural world, not just trust in technology (which some people inherently distrust).
Why Reverse Engineering Google Hummingbird provides Startlingly Accurate Topic Analysis
If we look at top, authoritative pages in the SERP for a parent topic there are many ranking signals which help a page perform well. Some of the most important include:
- Links to the page and domain
- Low bounce rate and high engagement rate
- Quality content (determined by Google’s machine learning and Hummingbird algorithm)
- Speed, time to load page
- Age and clean history of domain name
- Website not “over-optimized” as judged by Google’s Penguin filter
Any of the above could affect a page’s chance of showing in groups we compare side by side with my topic analysis techniques. However, the bias won’t push out sites with particular characteristics related to what we care about, “quality content” (the overly simplistic term Google uses, a term which is about their guidelines built into the Hummingbird algorithm).
Strong linking (#1) to a page shows it’s gotten “votes of confidence.” Good: in addition to Google finding relevance, humans gave a thumbs up. An “over-optimized” (#6) page may have less natural, high-quality text about a topic. Time to load (#4) won’t bias whether the page has “quality content.” It’s a technical quality signal, but if a site is doing a poor job with technical implementation, perhaps they are doing poorly in other respects: writing about the topic we searched on, for example.
None of these ranking signals bias our research away from finding related topics. With the other phrases ranked from pages we use to find co-occurring phrases, there must be semantic relevance, otherwise Google wouldn’t send traffic. Right? From my experience in all instances of comparing what Google ranks tops for parent topics (the search phrases) these techniques always produce very relevant results.
The beauty of “co-occurring” is most of these other phrases do not appear more than and few times. Therefor, they are very distant in association and we know not to focus on them. Sometimes the other ranking words are picked up by Google because the website itself it so authoritative that any remotely related phrase gets ranked high if it’s not too competitive. Or, they are relevant as much to other pages on the website as to the topic of the page. No problem, our technique pushes these words out of the group with significant numbers of co-occurrences.
Remember, Google’s goal is to answer searchers’ questions. It ranks the other terms on a page if they are relevant based on its machine learning. If “Wizard of Oz” is not appearing on other authority web pages, because it is irrelevant to your parent topic, it will not send people to an irrelevant page and domain that’s not about Oz.
A popular website about the “The Incas” will be ranked in part because it has the linking “vote of confidence.” What are the chances “Wizard of Oz” will appear on both the National Geographic and Wikipedia page about the people that once inhabited the Andes? Very, very low. If it appears, there’s relevance. You may be an expert on the Inca people, but what if many websites are discussing brand new breaking news: “The Wizard of Oz” was just found to be inspired entirely by ancient Inca folklore? If you missed the news, the topic analysis finds it for you.