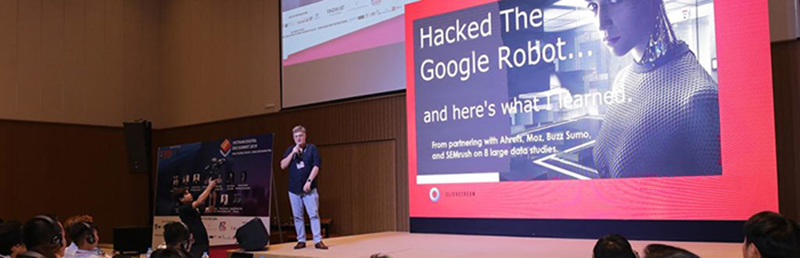
This post uses older data than the final write-up I did on Search Engine Journal. The purpose here is to demonstrate how Tableau Public is used with publically available SEO data. Please focus on these data visualizations as use cases not on whether this data is still relevant in 2024 and 2025. The ideas are entirely relevant today. The findings may not be.
Go directly to the data viz! Click Here.
Websites in “Your Money Your Life” (YMYL) categories saw a roller-coaster ride in the SERPs over the last two years. One way of understanding the changes is that Google tries to be more cautious in ranking pages related to personal health. This might be especially true if the topics lack wide consensus, are controversial or have an out-sized impact on personal health choices. Google judges on-site content, in part, via their machine learning and natural language processing (NLP) algorithms.
To investigate, we compared web pages that cover health-sensitive topics vs. non-health pages that do not fall into the now-infamous YMYL category. ClickStream partnered with Surfer SEO to collect data for this study.
Here are the most critical findings from the study:
- Google increases its sensitivity to on-page factors for health-sensitive topics, especially those classified as “Your Money or Your Life” (YMYL).
- To achieve high rankings for YMYL pages, content needs to be thorough and holistic compared to pages on less critical topics.
- A high Content Score from Surfer SEO is a stronger predictor of ranking success than any single on-page ranking factor.
- Covering a parent keyword comprehensively, including related topics, is the most influential on-page ranking factor. This approach outweighs the importance of Title tags and H1 elements. Keep in mind, though, the analysis focused on low to medium search volume keywords.
- Informational intent keywords demand stronger on-page optimization than transactional or buying-intent keywords.
Methods
We looked at the top 30 results on Google SERP for 8,460 keywords. That resulted in over 200,000 ranking URLs. We analyzed a randomized 190,824 final SERP results.
Most on-page rank studies require developing or re-using a custom crawler to gather webpage data specifically for “studies.” Surfer’s crawler and algorithm, however, analyze the information it collects from webpages and SERPs hundreds of times a day. This is at the heart of their tool’s secret sauce and success. In a sense, every time Surfer SEO’s public-facing tool analyzes SERPs for a user’s keyword submission, they do a mini-rank study on the top results for that keyword.
The keywords came from NLP-related search terms related to:
- board games
- musical instruments
- vapes
- outdoor lighting
- patio seating
- pen scanners
- ski bags
- CBD
- software
- dangerous/prohibited/banned products from Amazon
CBD and vape keywords are banned from Google ads. The FDA and others consider muscle building and weight loss as two of the riskiest health-related categories on Amazon. We chose the other categories because they are near poster children of innocuous niches.
Group Total URLs
Health-related | 28,087 |
Non-health related | 97,288 |
Dangerous/Prohibited/Banned | 65,449 |
Total | 190,824 |
Most SEOs don’t work for the top 50 largest websites, and we want results to help everyone. We chose keywords with monthly searches that were mostly below 1000 searches. These are siloed keywords from niches. The narrow niches also let us cluster topics that are very much not YMYL vs. those that are. Lastly, niche clusters let us look for new sector analysis techniques for SEO .
The “Dangerous/Prohibited/Banned” keywords were manually pulled and appended from Amazon’s lengthy list on their Seller Central page.
Adding Multiple-Variable Filters To See Results Beyond Two Dimensions
The data viz below let you adjust sliders and click on data points to understand better how relationships in the study are multi-dimensional. Google has are over 200 rank signals. Could someone build a machine to dial up or down each signal to predict better how to reach their SEO goals? Nope. However, adding at least a third dimension to our most essential results makes for some very interesting, granular analysis. Further, I created interactive data viz by category as a use case.
Using Data Visualizations for Niche and Industry Sector SEO
We’ve all seen metrics in SEO tools for “competitors,” but they are static measures. What if you find all the main keywords you want your website to rank for, and then see how other domains perform on, for example, comprehensive topic coverage? You can download the data with CSVs and use data visualization apps like Tableau Public, which I use here.
Note how similar websites get similar content scores. SEOs love to see metrics about competitors like ranking keywords, estimated monthly traffic, and number of linking domains. These are all super important. Seeing the individual’s keyword terms closely related to a domain is how we judge “competitors.”
I’ve always been fascinated by taking that to the next level. How do we look at entire sectors based on how keywords are “scored” by Google? Backward analyzing Google SERP gives those insights. Much of this analysis moves out of the SEO realm and into the business analysis realm. It’s also super helpful in evaluating niches to invest in for affiliate or eCommerce websites. Links might tell us a particular sector is overly competitive, but what about content quality? If we can evaluate large groups of keywords for different niches.
Hope you learned something new! We are planning out our next study and would love to get your feedback on Twitter.
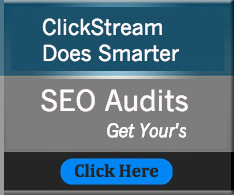
More About Correlations and Measurements in the Study
Niches were chosen because I wanted domains with multiple URLs to appear in our study. This was important to get a lot of eCommerce sites that are “specialty” oriented, as most non-mega e-commerce sites are. Most data studies do not look at how a group of URLs from one domain tell a story. The keywords they use are so randomized that the mega websites have the vast majority of URLs in results.
The narrow topics also meant fewer keywords with extremes in ranking competition. Many rank studies use a preponderance of keywords with over 40,000 monthly searches, but most SEOs don’t work for websites that can rank top 10 for those. There is a bias in this study toward less competitive keywords in our study, and I didn’t look at Google keyword search volume, just the volume on Amazon. Our keywords did not have less than 10 monthly searches on Amazon per month (via JungleScout) and given that I appended words to them, their actual search volume in Google would be less than 10 a month in many cases.
The “dangerous/prohibited/banned” group was excluded from most comparisons of health vs. non-health. Many of these were very esoteric topics or Amazon needed 6-10 words to describe them.
We used the Spearman rank-order correlation to examine data. Spearman is a formula that calculates the correlation between two variables. The correlation is measured from -1 to 1. A correlation coefficient that is 1 or -1 would mean that there is a strong monotonic relationship between the two variables. The reason why Spearman’s is used instead of Pearson is because of the nature of Google Search Results; they are ranked by importance, in decreasing order. Spearman’s correlation compares the ranks of two datasets, which fits our goal better than Pearson’s. We used .05 as our level of correlation confidence. This is the same confidence level I used when overseeing the 1 Million Search Results data study for Brian Dean in 2016.
When I show a correlation of .08, it suggests a ranking signal that is twice as powerful as another ranking signal measure of .04. Greater than .05 is a positive correlation, and less than .05 has no correlation. Correlations range from .05 – .05. A negative correlation shows it is causing the direct variable number to go down.
Many of the domains in the study are from outlier or niche topics or are small because of little time and money put into them. That is, first and foremost, why they don’t rank well. That is also why we must look for “controls” that might show that two domains had the same amount of time, web dev/design savvy-ness, and money put into them, BUT they are, for example, health vs. non-health topics.